Missing Values in Large Datasets
- IntelData Pty Ltd | Asset Management and Business
- Jan 1, 2016
- 2 min read
The most problematic of data problems are the missing data values. Null or empty cells are the most common representations of missing values.
Missing values in the datasets are the results of many causes:
Data entry errors
Unknown values due to loss of data. These could be the result of data corruption, overwriting the database tables, problems in data migrations
The data was not collected in the first place because of data collection limitations or human error
The data was deliberately withheld during data collection, such as with surveys and questionnaires
Datasets might contain missing values because of many other reasons.
Different Types of Missing
Figure 1 shows the types of missing values in the datasets.
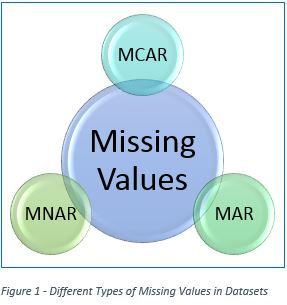
[endif]--These abbreviations are often used by statisticians. Understanding the meaning of these different types of missing values can affect the method(s) by which the missing values are imputed.
MCAR
This is the abbreviation for Missing Completely at Random.
MCAR means that the there is no way to determine what the missing value should have been. Random imputation methods as well as any other method work for MCARs.
MAR
This is the abbreviation for Missing at Random. While this seems to be the same as MCAR, MAR implies a conditional relationship between the missing value and other variables. The missing value itself is not known and cannot be known but it is missing because of another observed value.
For example, if answering “Yes” to question 10 on a survey means one does not answer question 11, the reason question 11 is missing is not random, but the answer to question 11 would have been, had it been answered cannot be known.
MNAR
This is the abbreviation for Missing Not at Random. In cases of MNSR the missing values can be inferred by the mere fact that the value is missing. For example, a responder in a survey may not provide information about criminal record if they have one, whereas those without a criminal record would report “no records”.
In case the missing value is suspected to be MNAR, it is highly recommended that the it should not be imputed with constants or at random if possible because these values will no reflect well what the missing value should have been.
Reference
Abbott, Dean. Applied Predictive Analytics. s.l. : John Wiley & Sons, Inc., 2014.
![endif]--
Comments